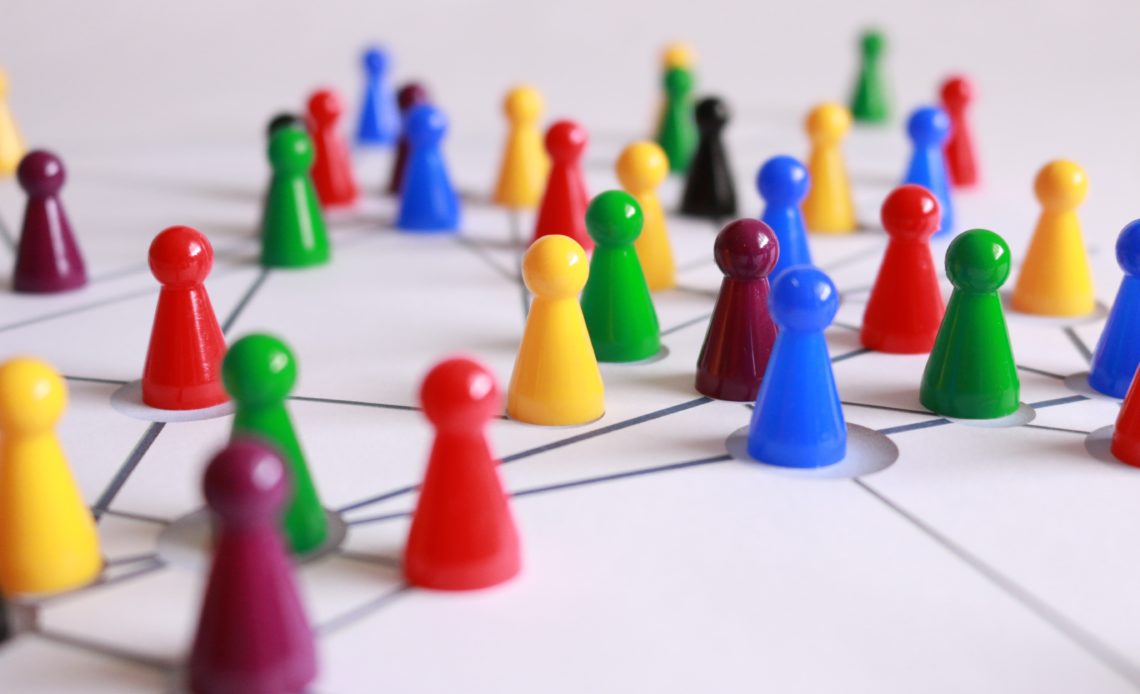
Vertical disintegration of network elements into smaller components is preparing the ground for accelerated innovation–vertical integration created barriers to entry for new players. Conversely, smaller companies now have room to bring specialized network solutions to the market with the splintering of monolithic Radio Access Network (RAN) solutions.
Increasing recourse to standard off-the-shelf hardware for RAN and their evolution into Software-Defined Radio (SDR) has opened the way for a diversity of open software, such as RAN Intelligence Controller (RIC), to provide innovative solutions. Standardized interfaces and their interoperability with APIs pave the way for agile solutions development to adapt to market changes.
RIC, a crucial component of the Open RAN architecture, adds programmability to optimize RAN resources to meet performance expectations of different uses cases. It exercises granular control over RAN resources by changing the real-time operating metrics or adjusting the non-real-time variables such as machine learning models, policies, and configurations.
Within the RIC framework, operational artificial intelligence controls network performance with applications in real-time (x-Apps) to achieve service assurance goals by correcting anomalies in operating parameters and other means.
Furthermore, the radio network elements (virtual versions of the centralized unit (CU), distributed unit (DU), and radio unit (RU)) feed data into a repository for analysis in non-real-time (r-Apps). The data is analyzed to inform changes in analytical models, policies, and configurations to change the parameters that influence network performance.
By leveraging xApps and rApps, RIC supports a number of use cases, including traffic steering based on network conditions, QoE optimization based on application performance, massive MIMO optimization to enhance network quality, sharing of RAN resources, end-to-end network slicing, and more.
An example of an x-App, driven by AI, is predicting the data streams from various applications and directing them to flow without encountering bottlenecks by multiplexing for their smooth flow. By contrast, a non-real-time application would take decisions on cell-splitting to realize the maximum throughput. For push-to-talk applications for emergency response, x-Apps provide consistent quality of service. There will also be third-party applications available on network app stores, such as navigating a drone’s flight path.
Innovations with RAN Intelligent Controller platform
A Vodafone-led alliance of companies, with participation from Cohere Technologies, VMware, Capgemini Engineering, Intel, and Telecom Infra Project (TIP), demonstrated a doubling of the capacity of a Multi-User MIMO (MU-MIMO) 5G cell site using a programmable RAN Intelligent Controller (RIC) with contributions from multiple vendors. The Cohere Spectrum Multiplier MU-MIMO scheduler used in the trial doubles the bandwidth of 700MHz IoT networks using traditional MIMO antennas. In mid-band spectrums, it is capable of capacity gains of 4 to 5x.
Deutsche Telekom has deployed massive MIMO (mMIMO) radio units using O-RAN open fronthaul interfaces to connect to the virtualized RAN software at ‘O-RAN Town’ deployment in Neubrandenburg, Germany. It is a multi-vendor open RAN network, with equipment from Dell, Fujitsu, Intel, Mavenir, NEC, and Supermicro, across 25 sites. The first few are already deployed with automation to manage the complexity.
Nokia collaborated with AT&T in New York City to test ‘xApps,’ at the edge of AT&T’s live 5G mmWave network on an Akraino-based Open Cloud Platform. The xApps contribute to enhancing spectrum efficiency and offer locational and use case-based customization. In addition, the RIC speeds up the execution of control functions by applications.
Programmability in Private 5G networks to manage dense traffic
Programmability of RAN is crucial to meet the performance of mission-critical enterprise applications. 5G private networks in highly dense traffic, from data-rich applications such as video inspection in factories, aerial vehicles, IoT, robots, concentrated at the edge with MEC, need to apportion network resources with exceptional precision. Additional complexities arise while directing data flows to several networks such as private LTE, Fixed Wireless, 4G/5G cellular wireless, and Wi-Fi 6 to meet the demands of applications; the risk of radio interference is ever-present.
The programmability and granularity of RAN Intelligence Controllers lend well to steer private cellular network traffic and optimize the performance of software-defined massive MIMO radios for indoor coverage.
Conclusions
A growing range of network needs, varying across customers’ application needs, will give rise to programmable network services that software and artificial intelligence can address. These services will demand a variety of performance attributes with associated trade-offs of cost. X-Apps and r-Apps will expand in numbers as they address a growing number of pain points.